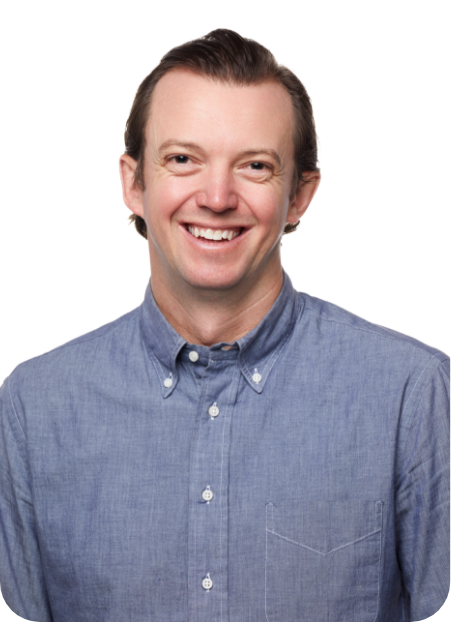
Graham Thompson
· 6 min read
Data Anonymization in AI: A Path Towards Ethical Machine Learning
Data is the new oil, lubricating the engines of Artificial Intelligence (AI) and Machine Learning (ML), ceaselessly driving us towards an era of automated enlightenment. However, as we guzzle down this invaluable resource, there's a lingering side effect of ethical indigestion. Reality hits hard when we realize that amidst this data binge, we might be inadvertently serving up generous portions of personal or sensitive information on the platter of AI/ML training and applications.
Data Anonymization
Data Anonymization is like a digital disguise, allowing data to maintain its essence while shedding identifiable traits. Essentially, it transforms data into a version where individual identification becomes impossible, yet the data stays relevant for analysis or operational use.
The goal? To strike a balance between preserving privacy and retaining data utility. In a data-driven world, anonymization navigates the tightrope of ethical data use, enabling organizations to extract valuable insights without risking identity exposure.
Data anonymization is designed to align data utility and privacy protection, ensuring a balanced approach in your operational environment.
The Intersection of Data Anonymization and AI/ML
You can’t look anywhere these days without encountering Artificial Intelligence (AI) and Machine Learning (ML). They are pivotal forces, with data serving as the critical fuel powering these technologies. However, utilizing personal or sensitive data elicits substantial ethical and privacy concerns. Data anonymization emerges as a crucial methodology to mitigate these concerns, transforming data into a form that safeguards individual identities while preserving its utility for AI/ML applications.
Consider the healthcare sector, which is increasingly leveraging AI-powered diagnostic tools. The deployment of these tools necessitates extensive training on patient data. The journey towards harnessing AI for improved patient outcomes, however, is riddled with privacy hurdles. The potential exposure of sensitive patient information is a significant deterrent that could thwart progress. Data anonymization comes to the forefront as a vital solution, converting sensitive data into a sanitized version devoid of identifiable information yet retaining its intrinsic value. This transformation allows the healthcare system to progress with training AI models without infringing on the privacy rights of individuals.
In the context of AI/ML model training, anonymized data plays a crucial role akin to a well-designed shield, protecting individual identities while allowing the models to learn and evolve. The AI/ML algorithms, devoid of any knowledge about the identities embedded within the data, continue to learn from the rich, meaningful information encapsulated in the anonymized data.
The technical dimension of data anonymization in AI/ML is a nuanced landscape. Various anonymization techniques like k-anonymity, l-diversity, and t-closeness ensure that the AI/ML models are fed with rich, meaningful data sans the risk of privacy infringement. Each of these techniques offers a unique approach to preserving privacy while maintaining data utility, a balance that's crucial for the practical application of AI/ML.
Consider also the financial services sector, where AI/ML applications are instrumental in detecting fraudulent transactions. The stakes are high, with a dual imperative: protecting customers from fraud while preserving their privacy. Data anonymization orchestrates a viable solution, facilitating the training of robust fraud detection models on datasets where sensitive information is cloaked, yet the patterns indicative of fraud are distinctly discernible.
Similarly, in the social media sphere, AI/ML models are employed to sift through the vast swathes of user-generated content to filter out harmful material. The user-generated data is a rich resource but is laden with privacy concerns. Data anonymization ensures a veil of privacy while enabling the AI/ML models to learn to differentiate between benign and malignant content diligently.
As we navigate through the diverse landscapes where AI/ML is making significant inroads, the importance of data anonymization in promoting ethical AI/ML practices becomes abundantly clear. The narrative of progress in AI/ML is intertwined with the narrative of privacy preservation, with data anonymization as the link bridging them.
Techniques of Data Anonymization in AI/ML
Data Anonymization in AI/ML is a craft honed with sophisticated techniques. Here's a deep dive into the critical techniques tailored for the AI/ML tapestry:
-
k-Anonymity:
- Definition: A dataset is said to have k-anonymity if the information for each person contained in the release cannot be distinguished from at least k-1 individuals whose information also appears in the release.
- Advantages: Provides a robust shield against identity disclosure.
- Challenges: This may lead to loss of data utility and does not protect against attribute disclosure.
-
l-Diversity:
- Definitio:n An extension of k-anonymity, l-diversity ensures that within each anonymized group, there are at least "l" diverse values for the sensitive attributes.
- Advantages: Offers a fortified defense against attribute disclosure.
- Challenges: Higher computational complexity and potential information loss.
-
t-Closeness:
- Definition: A further extension, t-closeness demands that the distribution of a sensitive attribute in any anonymized group is close to the distribution of the attribute in the overall table within a threshold t.
- Advantages: Addresses the shortcomings of l-diversity, providing a more balanced privacy-utility trade-off.
- Challenges: Computational complexity and the need for a well-defined distance metric.
-
Differential Privacy:
- Definition: A technique that ensures the disclosure of statistical information about a dataset does not compromise the privacy of individuals within the dataset.
- Advantages: Provides strong privacy guarantees, suitable for dynamic datasets.
- Challenges: It may inject noise into the data, potentially impacting data utility and requiring careful parameter tuning.
Each of these techniques unveils a pathway to harness the potential of AI/ML while navigating the labyrinth of privacy concerns. The choice of technique hinges on the unique privacy-utility landscape of each AI/ML endeavor, demanding an evaluation to ensure privacy without stifling the project's goal.
Benefits and Challenges
It isn’t all upside to making sure your AI/ML algorithms are trained with privacy in mind. Let’s explore:
Benefits:
-
Privacy Preservation:
- Data anonymization acts as a defensive layer, safeguarding individual privacy amidst the data deluge essential for AI/ML projects.
-
Ethical Compliance:
- Aligns AI/ML projects with prevailing legal and ethical standards, fortifying the foundation of ethical AI and ML applications.
-
Public Trust:
- Fosters a climate of trust, indispensable for public acceptance and successful deployment of AI/ML technologies.
Challenges:
-
Model Accuracy:
- The veil of anonymization, while protecting privacy, could blur the sharpness of data, affecting model accuracy.
-
Computational Complexity:
- The computational heft required for implementing robust anonymization techniques could pose challenges, especially in resource-constrained settings.
Future Trends
Exploring upcoming trends that signify a maturation of anonymization techniques and a broader understanding of privacy challenges. From more refined anonymization methods to integrating holistic privacy frameworks and cross-disciplinary initiatives to community-driven standards, the horizon is ripe with promise for fostering a privacy-centric AI/ML ecosystem.
Some trends we expect advancement in:
-
Advanced Anonymization Techniques:
- The future promises more sophisticated anonymization techniques, enhancing the privacy-utility trade-off in AI/ML projects.
-
Holistic Privacy Frameworks:
- Integrating data anonymization within broader, holistic privacy frameworks is a burgeoning trend, leading to a more comprehensive approach to privacy preservation in AI/ML.
-
Cross-disciplinary Approaches:
- The confluence of insights from law, ethics, and technology catalyzes innovative data anonymization practices, forging a cross-disciplinary tool to combat privacy challenges in AI/ML.
-
Privacy-Preserving AI/ML Frameworks:
- The emergence of frameworks designed to embed privacy preservation within the core of AI/ML models, facilitating seamless integration of data anonymization practices.
-
Educational Initiatives:
- A spurt in educational initiatives aimed at fostering a deeper understanding and skill development in data anonymization, empowering the next generation of AI/ML practitioners to build privacy-centric AI/ML models.
-
Community-Driven Standards:
- The crafting of community-driven standards and best practices for data anonymization sets the stage for a collective stride toward ethical AI/ML practices.
-
Government and Industry Collaborations:
- Enhanced collaborations between governmental bodies and industry stakeholders, driving forward the agenda of ethical AI/ML through effective data anonymization practices.
Data anonymization is central to ethical AI/ML practices, enabling innovation while safeguarding privacy. The importance of ethical considerations in AI/ML projects is evident. We welcome you to explore data anonymization solutions offered by Privacy Dynamics to navigate the ethical landscape of AI/ML.
To explore these ideas further, check out the pages linked below. Gain insights on data anonymization for a test environment, learn how to de-risk data sharing, and understand why businesses need to anonymize data.
To learn more about Privacy Dynamics and how we can help you unlock production data while reducing privacy risk, give us a shout or sign up for a free trial at https://signup.privacydynamics.io - We’re looking forward to helping you!